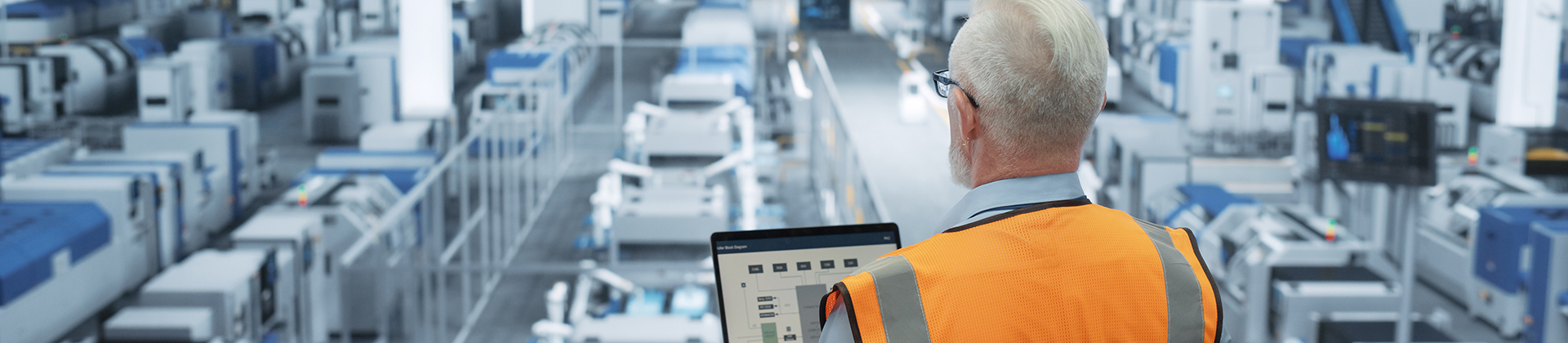
Increase uptime and efficiency in your systems
In today's fast-paced industrial landscape, the importance of proactive maintenance strategies cannot be understated. Predictive maintenance, enabled by intelligent drive edge computing and condition monitoring, has emerged as a powerful tool to optimize equipment performance, increase uptime, and reduce maintenance costs. In this article, we will explore the concept of predictive maintenance in relation to condition monitoring and highlight its numerous advantages in terms of economic efficiency, equipment performance, and cost savings.
Advantages of predictive maintenance condition monitoring
Increased economic efficiency
By continually collecting and monitoring data, predictive maintenance ensures that equipment issues are identified before they escalate, minimizing the risk of downtime. This proactive approach enhances economic efficiency by preventing costly unplanned shutdowns and reducing overall maintenance expenses.
Higher uptime
The constant monitoring of data allows for early identification of any changes or abnormalities in equipment performance. By addressing potential issues before they lead to failure, predictive maintenance maximizes equipment uptime, ensuring smooth operations and minimizing disruptions.
Extended machine/equipment lifetime
Through timely maintenance and addressing potential issues proactively, predictive maintenance helps to optimize the performance and lifespan of machines and equipment. By identifying and rectifying issues before they cause severe damage, the need for expensive repairs or premature replacement is significantly reduced.
Optimal preventive maintenance timing
Predictive maintenance utilizes condition monitoring data to determine the best point in time for maintenance activities. By analyzing the health state of components and following the degradation curve, maintenance can be triggered before functional failure occurs. This approach ensures that preventive maintenance activities are carried out when they are most needed, preventing unnecessary downtime and reducing costs associated with reactive repairs.
Edge analytics and machine learning for better machine performance
The collected data from condition monitoring provides valuable insights into the performance of machines and equipment. By analyzing this data using edge analytics and applying machine learning algorithms, manufacturers can identify patterns, trends, and potential areas for improvement. This information can be used to optimize machine efficiency, resulting in better overall performance and increased productivity.
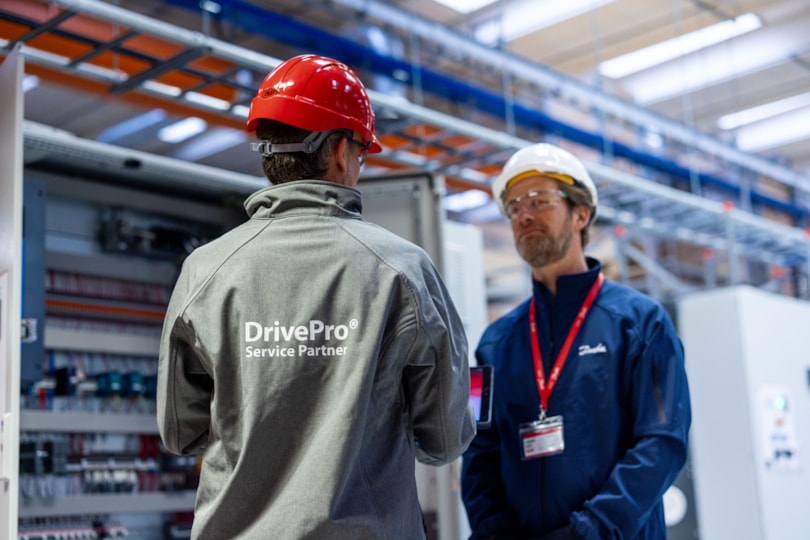
Don’t let your CAPEX slip away, invest in savings that don’t stop at the sale!
In today's competitive landscape, it’s crucial to make the most of your CAPEX. Invest in a proactive maintenance strategy that goes beyond just the sale. By prioritizing early detection and consistent upkeep, you’re not only safeguarding your investments but also driving long-term savings.
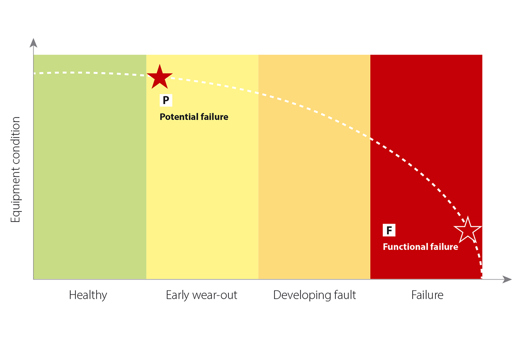
Significant savings potential of condition-based maintenance (CBM)
A study commissioned by the European Commission highlights the significant savings that can be achieved through a properly functioning condition-based maintenance (CBM) program. The study estimates savings of 8-12% over traditional preventive maintenance schemes. Additional benefits reported include a reduction in maintenance costs by 14-30%, downtime by 20-45%, breakdowns by 70-75%, and an improvement in production by 15-25%.The algorithm compares the actual cavitation signature against user-defined threshold levels. If the values exceed the set thresholds for a predefined time, the event is flagged as cavitation.
Furthermore, repair costs for failed assets are typically 50% higher than if the problem had been addressed prior to failure. Reports from companies like Fusheng in the compressor industry indicate that timely repairs resulted in a 15% reduction in mean time to repair (MTTR) and a 20% increase in the first-time fix rate.
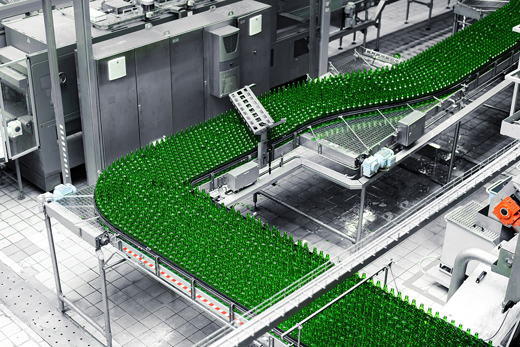
How analytics are leveraged in predictive maintenance
Predictive maintenance relies on advanced analytics to leverage the collected data effectively. This includes:
- analyzing component lifetime and fault information
- implementing condition-based maintenance strategies
- establishing baseline information for comparison
- utilizing machine learning algorithms to identify patterns and make accurate predictions
These analytics enable manufacturers to prevent unexpected issues, optimize availability, reduce wear and tear effects, extend equipment lifetimes, and create predictable long-term cost savings through tailored maintenance plans.
Maximize productivity with intelligent edge computing
Predictive maintenance, enabled by intelligent drive edge computing and condition-based monitoring, helps manufacturers achieve optimal equipment performance, increased uptime, and cost savings. By systematically ensuring the optimal condition of machinery and addressing potential issues before they escalate, businesses can avoid unexpected downtime, extend equipment lifetimes, and maximize overall productivity. Embracing predictive maintenance not only reduces complexity but also provides actionable insights that take the guesswork out of maintaining equipment, ensuring a competitive edge in today's rapidly evolving industrial landscape.
Highlighted products
Danfoss products using condition-based monitoring
-
if (isSmallPicture) {
; } else if (isBigColumns) { } else { }VLT® Refrigeration Drive FC 103FC103 is dedicated to controlling compressors, pumps and fans for significant energy savings in refrigeration plants.
-
if (isSmallPicture) {
; } else if (isBigColumns) { } else { }VLT® AQUA Drive FC 202Maximum energy efficiency for both water /wastewater and Industrial pump/fan applications
-
if (isSmallPicture) {
; } else if (isBigColumns) { } else { }VLT® AutomationDrive FC 301 / FC 302The VLT® AutomationDrive FC301 / FC302 is designed for variable speed control of all asynchronous motors and permanent magnet motors. It comes in a standard version (FC301) and an advanced high dynamic version (FC302) with additional functionalities.
-
if (isSmallPicture) {
; } else if (isBigColumns) { } else { }VLT® HVAC Drive FC 102This tough and savvy FC102 drive enhances pump and fan applications in building management systems, and runs outdoors in most climates.
-
if (isSmallPicture) {
; } else if (isBigColumns) { } else { }VACON® 100 XA drive for indoor and outdoor applications that withstands high pressure and vibration levels, water, heat and dirt.
-
if (isSmallPicture) {
; } else if (isBigColumns) { } else { }VACON® 100 INDUSTRIALDrive modules and enclosed drives that are easily integrated into all major control systems.
-
if (isSmallPicture) {
; } else if (isBigColumns) { } else { }VACON® NXP Air CooledDesigned for a broad range of demanding applications, focusing on higher power sizes and system drives.
-
if (isSmallPicture) {
; } else if (isBigColumns) { } else { }VACON® 100 FLOWDedicated functionality that improves flow control and saves energy in industrial pump and fan applications.
-
if (isSmallPicture) {
; } else if (isBigColumns) { } else { }VACON® NXP System DriveConfigured and assembled to meet your needs whether you need to control one or several motors.
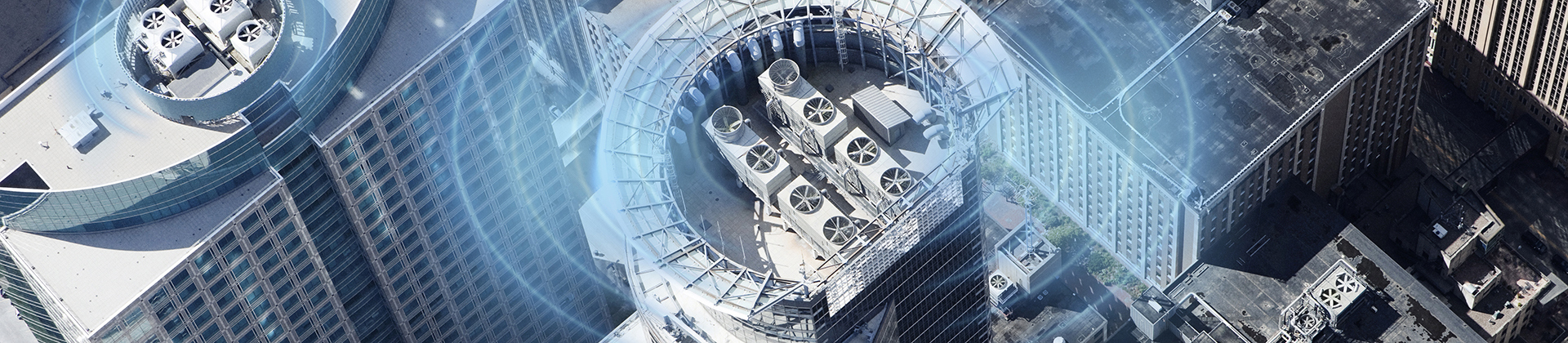
Danfoss first innovations
CBM has emerged from a history of Danfoss firsts in innovation. Danfoss drives differentiate from others in the market with intelligent functions embedded in the drive, to reduce the external components required.